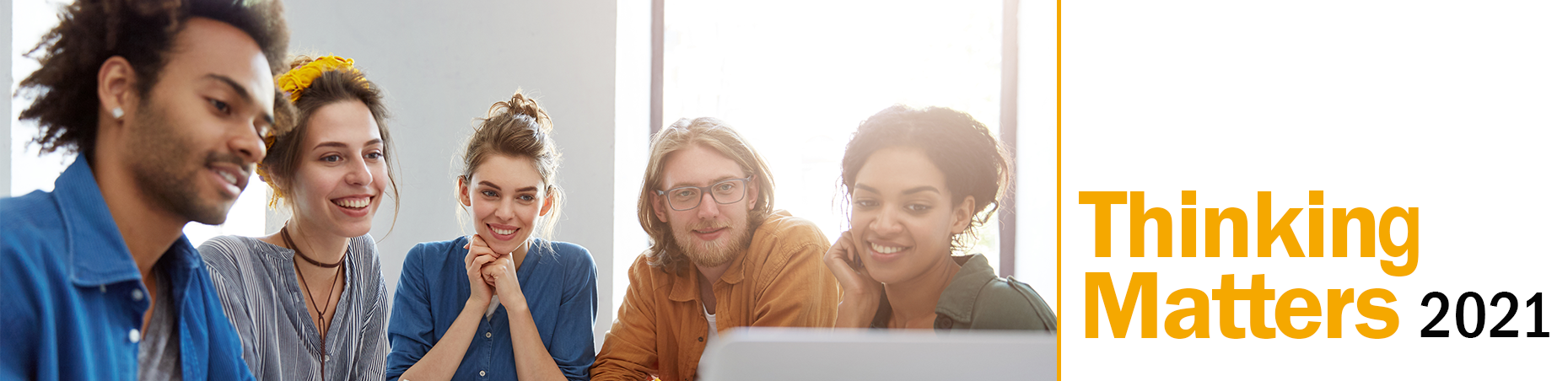
Loading...
Document Type
Oral Presentation
Department
Engineering
Faculty Mentor
Asheesh Lanba, PhD
Keywords
LATscan, Artificial Intelligenc, e Neural Network, UNET, RootPainter, Ilastik, Soybean Segmentation, Computer Vision
Abstract
Improvements in contemporary machine learning architectures have drastically increased the available tools in biological analysis, particularly in feature segmentation in 2D images and 3D models. A major obstacle for building machine learning architectures is associated with generating large amounts of training data, a process which requires significant time and processing resources. Recent software tools allow for an interactive training approach that show great promise in reducing the time and effort needed to generate training data. This study analyzes cross-sectional images of Soybean stems obtained via Laser Ablation Tomography (LATscan) using two open-source interactive machine-learning tools. Both software tools are trained to identify Xylem conduits depicted in the LATscan images, from which count and area data are collected. This resulting information is then compared to identify any possible bias in the algorithms and training methods. The results show effective creation of predictive models with a low amount of training data, thus presenting plant scientists with a powerful new tool for quantifying plant features that can be used for high impact phenotyping studies.
Interactive Machine Learning Methods for the Quantification of Vascular Features in Soybean Images Obtained via Laser Ablation Tomography (LATscan) - transcript
Open Access?
1
Interactive Machine Learning Methods for the Quantification of Vascular Features in Soybean Images Obtained via Laser Ablation Tomography (LATscan)
Improvements in contemporary machine learning architectures have drastically increased the available tools in biological analysis, particularly in feature segmentation in 2D images and 3D models. A major obstacle for building machine learning architectures is associated with generating large amounts of training data, a process which requires significant time and processing resources. Recent software tools allow for an interactive training approach that show great promise in reducing the time and effort needed to generate training data. This study analyzes cross-sectional images of Soybean stems obtained via Laser Ablation Tomography (LATscan) using two open-source interactive machine-learning tools. Both software tools are trained to identify Xylem conduits depicted in the LATscan images, from which count and area data are collected. This resulting information is then compared to identify any possible bias in the algorithms and training methods. The results show effective creation of predictive models with a low amount of training data, thus presenting plant scientists with a powerful new tool for quantifying plant features that can be used for high impact phenotyping studies.