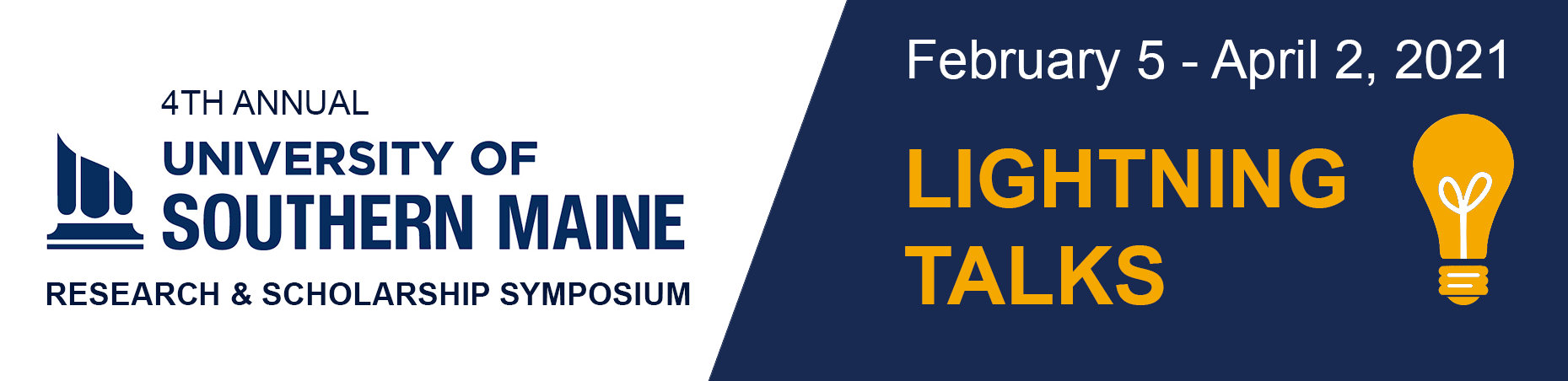
AI-Powered Analysis of Adverse Drug Events using Big Data Biomedical Literature and Deep Learning Neural Networks
Loading...
Start Date
26-3-2021 12:10 PM
End Date
26-3-2021 12:20 PM
Description
Adverse drug events (ADEs) as a set of detriments caused by medications, have led to additional medical cost, prolonged hospitalization, disability, and morbidity worldwide. The study of ADEs has been a longstanding topic in the medical literature, where several medical data sources, such as EHRs, medical case reports, and drug reviews have been widely employed to interpret the ADEs. In recent years, a vast number of scientific articles and health-related social media posts get published on a daily basis, however little is known about the contents associated with perusing adverse reactions resulting from medications. Of late, artificial neural networks have been increasingly used to discover hidden patterns and facts from massive and diverse clinical data sources, and they have demonstrated promising results in a variety of applications ranging from named entity recognition and clinical note understanding to computational phenotyping. In this presentation, we will discuss, from the computational side, the state-of-the-art big data deep neural network components we developed in the context of analyzing ADEs using biomedical literature. This presentation will consist of our recent findings and implementations, ongoing research and the future directions in using deep artificial neural networks to ADEs analysis.
AI-Powered Analysis of Adverse Drug Events - transcript
AI-Powered-Analysis-of-Adverse-Drug-Events_CC.srt (8 kB)
AI-Powered Analysis of Adverse Drug Events - Captions .srt file
AI-Powered Analysis of Adverse Drug Events using Big Data Biomedical Literature and Deep Learning Neural Networks
Adverse drug events (ADEs) as a set of detriments caused by medications, have led to additional medical cost, prolonged hospitalization, disability, and morbidity worldwide. The study of ADEs has been a longstanding topic in the medical literature, where several medical data sources, such as EHRs, medical case reports, and drug reviews have been widely employed to interpret the ADEs. In recent years, a vast number of scientific articles and health-related social media posts get published on a daily basis, however little is known about the contents associated with perusing adverse reactions resulting from medications. Of late, artificial neural networks have been increasingly used to discover hidden patterns and facts from massive and diverse clinical data sources, and they have demonstrated promising results in a variety of applications ranging from named entity recognition and clinical note understanding to computational phenotyping. In this presentation, we will discuss, from the computational side, the state-of-the-art big data deep neural network components we developed in the context of analyzing ADEs using biomedical literature. This presentation will consist of our recent findings and implementations, ongoing research and the future directions in using deep artificial neural networks to ADEs analysis.