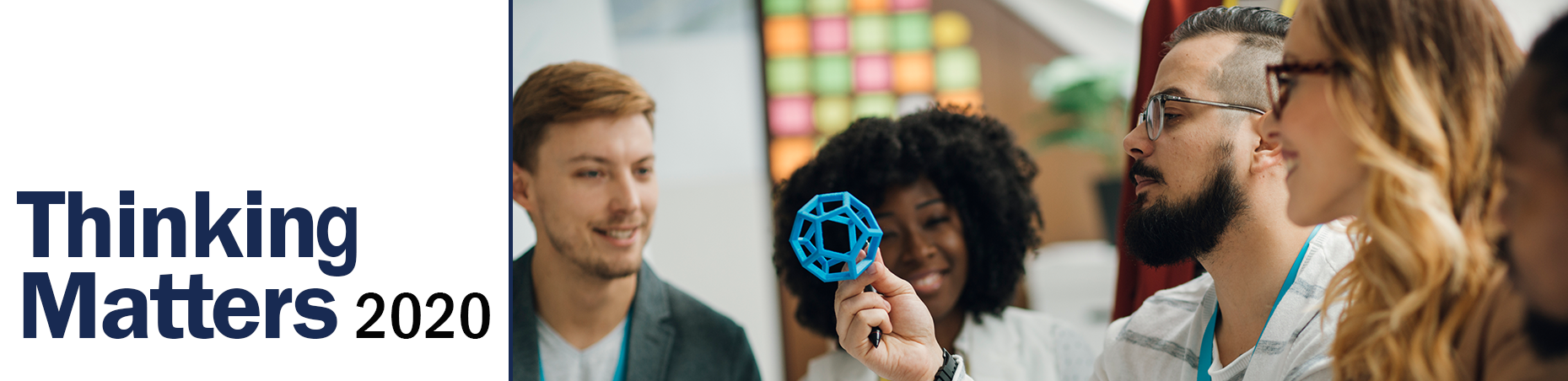
Document Type
Poster Session
Department
Biological Sciences
Faculty Mentor
Douglas Currie, PhD
Abstract
Through our research we hope to demonstrate the efficiency and accuracy of deep learning technology in automating the classification of arsenic exposure at the cellular level. Classification of arsenic exposure is currently a human task that requires visual inspection of the cellular structure to find abnormalities. This is a task that can be successfully automated with computer vision. Current research shows that models can successfully recognize the cellular morphology caused by varying degrees of arsenic exposure, and we are working to isolate the model training practices that optimize accurate predictions.
Our research involves comprehensive analysis of various model training methodologies, neural network architectures, and algorithms that can be applied towards automating this task. With a dataset of images provided by USM’s Biology department, we have worked with various crop sizes and preprocessing techniques to aid in the model’s learning process. This research is done to understand the techniques that promote the best learning at the cellular level and push the deep learning models further into toxin classification problems in more complex organisms.
It is hoped this research can inform others of the potential deep learning has in cellular and toxicological classification problems, as well as to automate the process of classifying arsenic exposure.
Open Access?
1
Arsenic Classification: Deep Learning Finding Toxin Exposure
Through our research we hope to demonstrate the efficiency and accuracy of deep learning technology in automating the classification of arsenic exposure at the cellular level. Classification of arsenic exposure is currently a human task that requires visual inspection of the cellular structure to find abnormalities. This is a task that can be successfully automated with computer vision. Current research shows that models can successfully recognize the cellular morphology caused by varying degrees of arsenic exposure, and we are working to isolate the model training practices that optimize accurate predictions.
Our research involves comprehensive analysis of various model training methodologies, neural network architectures, and algorithms that can be applied towards automating this task. With a dataset of images provided by USM’s Biology department, we have worked with various crop sizes and preprocessing techniques to aid in the model’s learning process. This research is done to understand the techniques that promote the best learning at the cellular level and push the deep learning models further into toxin classification problems in more complex organisms.
It is hoped this research can inform others of the potential deep learning has in cellular and toxicological classification problems, as well as to automate the process of classifying arsenic exposure.